Location Patterns of Housing Choice Voucher Households Between 2010 and 2020
Gretchen Armstrong, Social Science Analyst, Office of Policy Development and Research
Alexander Din, Social Science Analyst, Office of Policy Development and Research
Rae Winegardner, Social Science Analyst, Office of Policy Development and Research
Introduction
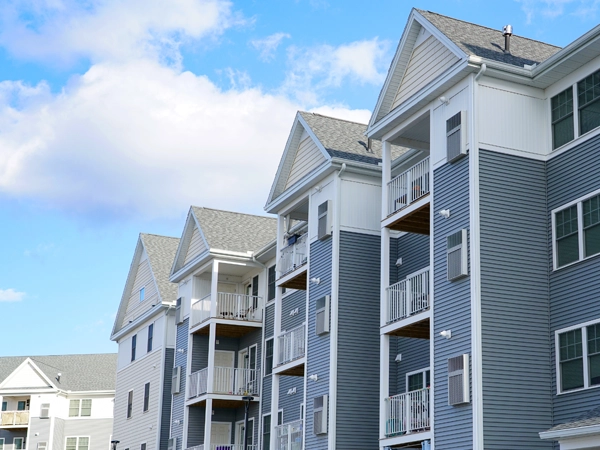
HUD began providing tenant-based rental assistance following the passage of the Housing and Community Development Act of 1974. This legislation represented a major shift in the way low-income rental assistance programs operated; for the first time, low-income households were able to use their assistance to seek rental housing in the private market. Previous low-income rental assistance programs, which were authorized through the Housing Act of 1937, operated fixed-site housing developments. By the 1970s, however, observers realized that the spatial concentration of poverty was negatively impacting those the program sought to help.
Tenant-based rental assistance, now called the Housing Choice Voucher (HCV) program, has inherent interests in neighborhood and locational outcomes for participating households, particularly those with children. The HCV program seeks to place households in higher-opportunity neighborhoods rather than areas of concentrated poverty, where many public housing developments were and still are located.
To mark the 50th anniversary of tenant-based rental assistance, Cityscape published a symposium reflecting on the program's history and future. Our article built on two previous reports examining location patterns of HCV households. The first report, which analyzed HCV households in 2000, found that HCV households living in the 50 most populous metropolitan statistical areas (MSAs) consumed a small share of both overall housing and affordable housing. The report also found that although most HCV households were in low- or moderate-poverty neighborhoods, approximately two in five HCV households lived in neighborhoods with poverty levels exceeding 20 percent, and Black and Hispanic program participants were more likely to live in high-poverty neighborhoods.
The second report compared nationwide trends in 2010 to those in the top 50 MSAs in 2000 and found that although the HCV program continued to consume a small share of affordable rental housing, the share of households living in high-poverty areas was increasing. The report also found that the share of HCV households living in HCV-dense census tracts had increased between 2000 and 2010. Both reports indicated that participant choice alone was not enough to achieve the program goal of spatial poverty deconcentration and suggested that addressing this goal required broader interventions and support systems that help participating tenants find housing.
Comparing Location Patterns of Housing Choice Voucher Households Between 2010 and 2020
In this third iteration of the HCV location report, covering 2010 and 2020, our purpose was to revisit the findings of the past reports, answer five questions about HCV households, and discuss a key policy change enacted during the study period: the introduction of Small Area Fair Market Rents (SAFMRs).
Our research questions were as follows:
- How have the demographics of HCV households changed?
- What share of affordable housing is consumed by HCV households?
- To what extent do HCV households concentrate in a few neighborhoods, and to what extent do they disperse widely across all neighborhoods?
- To what extent are HCV households located in low-poverty neighborhoods?
- What policy changes may affect where HCV households are located?
Our most notable finding was that the number of HCV households with an elderly or disabled head of household exceeded the number of HCV households with children. We also found that the share of HCV households living in neighborhoods with a high density of voucher holders increased from 2010 to 2020 and that a large share of HCV households still live in high-poverty neighborhoods.
Although HUD introduced SAFMRs during the study period, our analysis of them was limited because they were implemented too recently to have an observable impact on the 2020 data. As an increasing number of public housing agencies use SAMFRs, however, research into their impact on locational outcomes likely will be a key area of interest for a future HCV location report.
Demographic Changes
Our first key finding was that the demographics of HCV households changed during the previous decade (exhibit 1). Families with children are no longer the most common type of HCV household. As of 2020, elderly- or disabled-headed households constitute the most common HCV household structure. From 2010 to 2020, the number of elderly heads of households increased by nearly 10 percentage points, and the number of disabled heads of households decreased slightly. Our research did not examine whether this change is attributable to recent growth in set-aside vouchers targeted at specific populations; however, this question may be an area for future research. Non-Hispanic Black heads of households continue to represent the largest — and growing — racial/ethnic group of HCV households.
Exhibit 1
Reprinted from U.S. Department of Housing and Urban Development, 2024. "Location Patterns of Housing Choice Voucher Households Between 2010 and 2020," Cityscape 26: 2, Office of Policy Development and Research.
Housing Choice Voucher Density
Our second key finding was that, although HCV households continue to represent a very small share of U.S. households (2% of all households and 6% of renter households), HCV households increasingly tend to live in neighborhoods with a higher density of HCV households. This trend toward increased density was first observed from 2000 to 2010. We found that between 2010 and 2020, the share of HCV households living in areas with lower HCV density decreased, and the number of all HCV households living in census tracts with higher HCV density increased. (Exhibit 2).Exhibit 2
Reprinted from U.S. Department of Housing and Urban Development, 2024. "Location Patterns of Housing Choice Voucher Households Between 2010 and 2020," Cityscape 26: 2, Office of Policy Development and Research.
These changes in neighborhood HCV density between 2010 and 2020 were driven primarily by the many new voucher households added to the project-based voucher (PBV) program. Through the PBV program, public housing authorities (PHAs) enter into contracts directly with landlords, often for units in the same structure or otherwise close together (for example, an apartment building). Although PHAs added PBVs in all density categories, they were much more likely to have situated PBVs in areas of higher HCV density. PHAs use PBVs for multiple reasons. Some PBV sites are former public housing developments that have been converted into privately owned low-income properties through the Rental Assistance Demonstration (RAD) conversion program. In other instances, PHAs have chosen to use the PBV program because it addresses specific community housing needs, such as specialty vouchers for homeless veterans, that can locate housing near needed supportive services.
Neighborhood Poverty
Our third key finding was that the share of HCV households using tenant-based vouchers (TBVs, which make up the bulk of the HCV program) that are living in low-poverty areas versus high-poverty areas has not changed substantially since 2000. Although we observed a small increase in TBV households living in low-poverty areas between 2010 and 2020 (exhibit 3), this increase generally represents a return to the neighborhood poverty rates observed in 2000. Nationwide, 44 percent of TBV households still lived in high-poverty census tracts in 2020, including 7 percent that were living in areas of extreme poverty. As noted in previous reports, TBV households in urban areas were much more likely to live in high-poverty tracts than were those living in suburban areas. Racial and ethnic disparities in household location persisted. White TBV households were more likely to live in lower-poverty areas, and Black and Hispanic TBV households were more likely to live in areas of high or extremely high poverty.
Exhibit 3
Reprinted from U.S. Department of Housing and Urban Development, 2024. "Location Patterns of Housing Choice Voucher Households Between 2010 and 2020," Cityscape 26: 2, Office of Policy Development and Research.
Future Research
This article identified several key areas for future research. For example, HUD began requiring PHAs in 24 areas to use SAFMRs starting in 2018, and it designated an additional 41 areas to begin using SAFMRs in 2024. HUD calculates SAFMRs at the ZIP Code level in metropolitan areas, whereas the previously used Fair Market Rents (FMRs) set rents at the metropolitan area level. This new calculation method seeks to open up more high-opportunity neighborhoods to assisted households. Some research has analyzed the early effect of SAFMRs on the locational outcomes of HCV households; however, as more areas adopt SAFMRs, the effects of SAFMRs may be more significant for households entering the HCV program, so stakeholders should consider conducting more research. Although HUD currently uses both FMRs and SAFMRs, the agency may eventually evaluate whether to expand the use of SAFMRs nationwide.
Program demographics have changed, and the housing market itself has tightened. Our findings suggest the need for new policy interventions to continue to make progress toward HCV goals. As the population the HCV program serves continues to change, it will be useful to research the extent to which demographic changes are related to locational outcomes and whether different household types may require different supports. As rents have risen, particularly following the housing market changes brought on by the COVID-19 pandemic, households increasingly have become rent burdened, and PHAs are struggling to absorb higher rents. Future researchers must consider these program and housing market changes.
Deborah J. Devine, Robert W. Gray, Lester Rubin, and Lydia B. Taghavi. 2003. “Housing Choice Voucher Location Patterns: Implications for Participant and Neighborhood Welfare.” Washington, DC: U.S. Department of Housing and Urban Development, Office of Policy Development and Research. ×
Kirk McClure, Alex F. Schwartz, and Lydia B. Taghavi. 2015. “Housing Choice Voucher Location Patterns a Decade Later,” Housing Policy Debate 25:2, 215–33. ×
Ibid. ×
We limited this analysis of the HCV program and neighborhood poverty rates to the TBV program because TBV-assisted households seek out housing in different neighborhoods in the private rental market, whereas PBV-assisted households are assigned a unit by a PHA, and the PBV program operates more like a fixed-site program such as public housing. ×
McClure et al. 2015. ×