The FHA TOTAL Mortgage Algorithm: Providing Machine-Learning Analysis for Two Decades
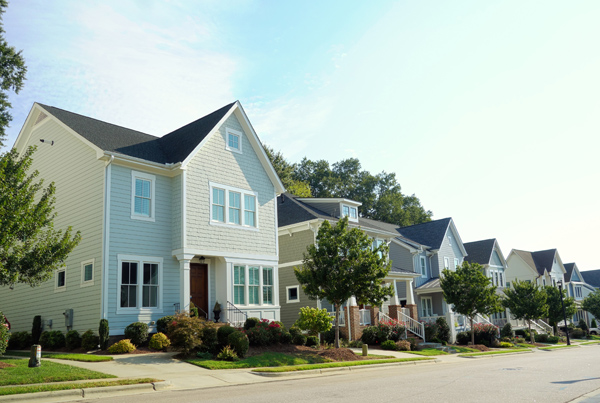
Adam Hoffberg and Bill Reeder
History of the FHA TOTAL Mortgage Algorithm
Since 1997, the Housing Finance Analysis Division of the Office of Policy Development and Research (PD&R) has provided independent subject-matter expertise to support the Federal Housing Administration (FHA) in its efforts to automate the approval of mortgage applications. Fannie Mae and Freddie Mac were developing proprietary credit risk scorecards for FHA lenders and servicers similar to those used for conventional mortgages, and PD&R initially was asked to help develop standards and validate the efficacy and fair lending impacts of these scorecards.
It soon became clear that FHA would need to develop its own scorecard that all FHA-approved lenders could use. Working with FHA, PD&R developed the Universal Scorecard for FHA Lending, which became known as the Technology Open to Approved Lenders (TOTAL) Scorecard. Fannie Mae and Freddie Mac rolled out TOTAL in 2004 and made it available to other FHA-qualified underwriting platforms.
TOTAL scores applicants’ credit risk by using their credit and mortgage application characteristics. These factors have demonstrated rigorous statistical association with mortgage repayment performance. FHA defines “poor performance” as loans that result in early defaults and become claims paid through FHA’s insurance fund. Typically, FHA automatically approves mortgage applications with scores above a certain threshold for insurance coverage. Applications with scores below the threshold may seek FHA endorsement through manual underwriting reviews.
PD&R partners with FHA and contractors to develop and maintain TOTAL, which is currently in its fourth version. PD&R provides FHA with technical and financial modeling services to support statutory and mission objectives in capital adequacy and access to credit. PD&R and FHA collaborate through a formal governance process to inform the development of policy.
PD&R uses cutting-edge techniques for forecasting mortgage performance, including the use of machine learning. PD&R’s rigorous “champion and challengers” process for validating TOTAL Scorecard specifications was used to evaluate more than 100 models in its last annual cycle.
PD&R not only tests TOTAL for compliance with Equal Credit Opportunity Act protections that support fair lending but also seeks specifications that affirmatively increase underserved populations’ access to credit. In addition, although TOTAL uses economic data to control for past economic effects when estimating the scorecard, it does not incorporate economics in the scoring of applications. TOTAL scoring neutralizes the effect of differential economic conditions and focuses on the characteristics of individual applicants and their mortgage terms.
PD&R has adopted this approach because FHA’s goal is to offer credit enhancement without regard to the business cycle. Including economics in TOTAL scoring likely would create procyclical effects; the availability of FHA credit enhancement would tighten during periods of economic contraction. This effect could further degrade macroeconomic performance and would result in applicants with similar credit profiles but at different points in the business cycle receiving materially different scores. Evidence of FHA’s countercyclical presence is the significant growth in FHA loan volumes in the wake of the 2008 financial crisis, when non-FHA lending tightened.
PD&R’s work helps FHA meet its National Housing Act target for capital adequacy of the Title II Single-Family mortgage programs. At the same time, FHA’s mission is to promote credit availability, especially for underserved populations. PD&R has successfully “squared the circle” by providing specifications that promote both capital adequacy and credit access.
TOTAL Scorecard’s Efficacy in Calculating Risk Levels
Since 2004, FHA has approved nearly 5.8 million loans through TOTAL with a sufficient history (known as “seasoning”) for a rigorous evaluation of how well TOTAL scores predict mortgage payment performance.
Within this population, approximately 450,000 loans (or 7.8%) have resulted in claims and meet other criteria for designation as adverse loan outcomes. These historical data quantify how effectively TOTAL ranks the credit risk of approved applicants. Data showing that adverse outcomes are concentrated at the lower range of TOTAL scores would suggest that TOTAL accurately ranks credit risk.
Since its inception, TOTAL has operated through periods with material variability in the quantity and credit performance of FHA-insured mortgages. (See figure 1.)
Figure 1
Only loans evaluated with the first three versions of TOTAL have sufficient seasoning to examine quantitatively. The 2008 financial crisis marked an important inflection point in the volume and credit quality of FHA endorsements. Endorsements before 2008 were of low volume and have demonstrated high rates of adverse outcomes. After 2008, FHA experienced high levels of demand, and those "books of business" have demonstrated lower rates of adverse outcomes.
As figure 2 illustrates, the first three versions of TOTAL have concentrations of adverse outcome loans at the lower ends of their score distributions. For example, at the 10th percentile, Version 1 captures 16 percent of the adverse outcome loans FHA experienced during the common seasoning period. Versions 2 and 3 evidence even stronger concentrations at 30 percent and 25 percent, respectively. By using a technique known as bootstrapping, we have concluded that the differences between the three concentrations are likely not attributable to chance.
Figure 2
An interesting implication of the concentration measures is that Version 2 of TOTAL performs better than Version 3. This finding seems counterintuitive considering that PD&R drew on its experience with Version 2 to develop Version 3. In fact, to assess the quality of Version 3, PD&R compared it to Version 2 using a common dataset of historical loan performance.
Differentiating the Effects of Scorecard Changes and Economics
Why does Version 2 outperform Version 3 in the concentration measure? The answer may lie in the economic and housing market conditions present during the different performance windows used to evaluate the two versions. Because TOTAL scoring neutralizes economic conditions, scorecard performance measured with concentration measures likely will vary with the conditions present when individual versions are used. Indeed, there is minimal overlap in the periods as one version replaces another. Furthermore, there was a high level of change in economic indicators during the period of analysis (2004 to 2019) with both strong and weak periods of growth in employment and house prices.
To differentiate the effects of scorecard versions and economics, we use logit regression, a multivariate technique that statistically controls for various factors. In this case, we model the association of whether a TOTAL-scored loan resulted in a “good” outcome with various explanatory variables. Logit models produce “odds ratios” that indicate whether an increase in a variable value increases the likelihood of a good outcome. An odds ratio above 1 indicates a positive association while a ratio less than 1 indicates a negative association.
The variables are summarized in table 1.
Variable | Description | Expected Effect on Dependent Variable |
Dependent Variable | ||
Good Loan Outcome | Binary indicator that a TOTAL-scored borrower experiences a good outcome, defined as avoiding an FHA insurance claim during the performance window. | Not applicable. |
Independent (Explanatory) Variables | ||
Borrower TOTAL Score | TOTAL scores normalized to the individual version’s overall median value. | Positive: a higher score should indicate better credit quality and performance. |
Version 2 Indicator | Binary value to isolate the effect of Version 2 on good outcomes compared with Version 1. | Positive: Version 2 should be a better predictor of good loan performance. |
Version 3 Indicator | Binary value to isolate the effect of Version 3 on good outcomes compared with Version 1. | Positive and larger than Version 2’s effect: Version 3 should improve upon Version 2. |
Unemployment | A measure of economic stress. | Negative: Higher unemployment should result in poorer loan performance. |
House price appreciation | The Federal Housing Finance Agency House Price Index. | Positive: Appreciation is associated with better loan performance. |
Mortgage rate changes after origination | The Freddie Mac Survey of Mortgage Rates. | Negative: Rising mortgage rates after origination impede the ability of borrowers and loan servicers to improve loan performance through changing loan terms as an interest rate modification or refinancing to reduce loan payments. |
The logit uses the following specifications of the economic variables:
- Unemployment: Highest unemployment rate over the first 4 years of seasoning.
- House price appreciation: Ratio of the national house price index at 4 years of loan seasoning to the index at the time of application. Values above 1 indicate positive appreciation.
- Mortgage rate changes after origination: The difference between the rate at the time of application and the average rate over the first 4 years of seasoning.
Table 2 shows the results this specification produced.
Variable | Odds Ratio* | Unit Measure |
TOTAL Score | 1.56 | 10% increase in score |
Version 2 Indicator | 2.24 | binary |
Version 3 Indicator | 2.96 | binary |
Highest unemployment rate over 4 years | 0.90 | 1 percentage point increase |
House price appreciation over 4 Years | 1.05 | 1 percentage point increase |
Difference between average mortgage rate over 4 years and rate at application | 0.78 | 1 percentage point increase |
Constant | > 0.00 |
AIC | 2,608,454 |
C-statistic | 0.82 |
n | 5,794,141 |
*All odds ratio estimates are significant at 1 percent. |
These results are consistent with theoretical expectations and exhibit a robust overall quality of fitness to the data. The odds ratio measures how much a change of 1 unit in an explanatory variable (defined above) increases the odds of statistical association with the outcome — in this case, a loan that does not go to claim (a "good outcome") during the performance window. An increase of 10 percent in the TOTAL score improves the odds of a good outcome by 56 percent. Using Version 2 of TOTAL more than doubles (a 124% increase) the odds of a good outcome, and using Version 3 almost triples the odds (a 196% increase). The latter is counter to the implication found in the concentration measures and suggests that Version 3 outperforms Version 2 when adjusting for the effects of economic conditions.
Conclusion
Overall, the FHA TOTAL Mortgage Algorithm has proven to be effective at calculating risk levels and has contributed to capital adequacy and credit access goals. Through machine learning and rigorous validation processes, PD&R has successfully developed a scorecard that promotes fair lending practices and enhances access to credit regardless of economic cycles. PD&R endeavors to continue improving TOTAL Scorecard performance.
These proprietary criteria are designed to focus on poor performance for situations in which the credit characteristics are more feasibly associated with credit performance and to deemphasize poor performance that is more significantly influenced by characteristics not directly associated with credit performance, such as job loss. ×
Another way to assess TOTAL's performance is by examining the mortgage performance of applicants who do not receive approvals. A high level of "good" mortgage performance among these applicants may suggest that TOTAL's risk ranking is suboptimal. Because most of these applicants will not take out an FHA-insured mortgage, however, we cannot conduct this analysis with FHA administrative data alone, and such an analysis presents methodological challenges in terms of what constitutes "good" mortgage performance. ×
For this evaluation, we bootstrapped 1,000 scenarios, with the middle 95 percent of distributions exhibiting no overlap.×
The C-statistic provides a measure of overall fitness for a logit scaled between 0.5 and 1.0. A measure of 0.5 indicates that the model is no better than a coin flip to forecast outcomes. A measure of 1.0 indicates a model that perfectly matches model-predicted outcomes with actual outcomes. ×